Table of Contents
Artificial Intelligence (AI) has come a long way in recent years, and one of the most intriguing and concerning phenomena within this field is AI hallucination. AI hallucination refers to the occurrence where large language models (LLMs) generate false information or facts that are not grounded in real data or events. These LLMs, such as GPT-3 and its successors, have made remarkable strides in generating text, translating languages, creating creative content, and providing informative responses. However, the potential for generating false or hallucinatory information is a critical concern in the world of AI. In this article, we will delve deeper into AI hallucination, exploring its causes, ways to prevent it, and the far-reaching implications it carries for society.
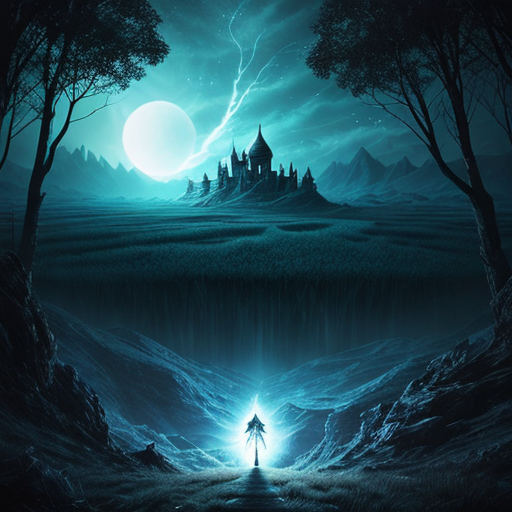
The Genesis of AI Hallucination
AI hallucination is not a result of mere happenstance; it arises from several key factors:
1. Insufficient or Low-Quality Training Data
The foundation of any LLM’s capabilities lies in the dataset it is trained on. If this dataset is too small or contains inaccurate and misleading information, the LLM may be more prone to generating hallucinations. Think of it as a painter trying to create a masterpiece with a limited palette of colors – the results may be incomplete or distorted.
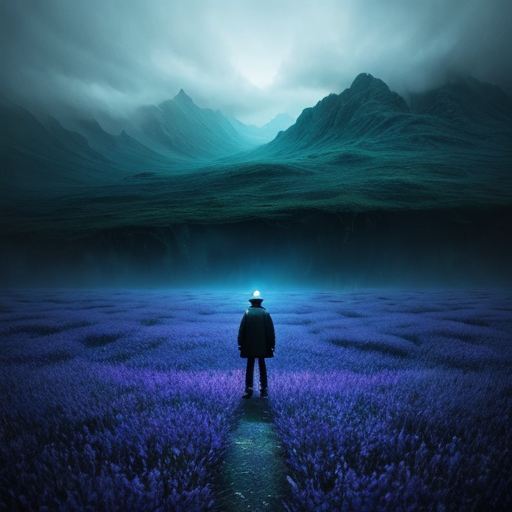
2. Overfitting
Overfitting is a perilous pitfall in AI training. It occurs when an LLM is trained on a dataset that is either too small or does not accurately represent the complexities of the real world. In such cases, the LLM may become overly specialized, learning the patterns within the dataset too thoroughly and failing to generalize to new, unseen data. This tendency to overfit can lead to the generation of outputs that are unreliable and hallucinatory.
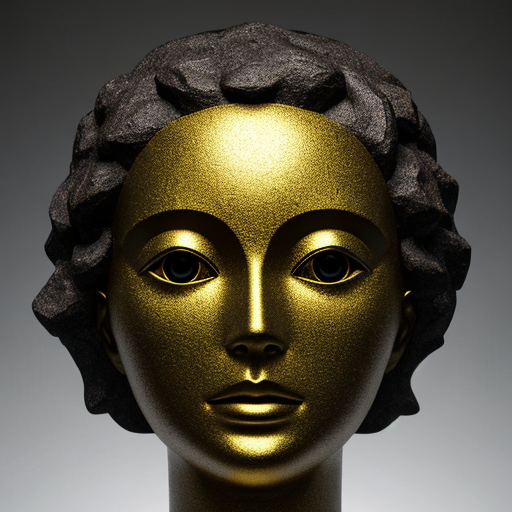
3. Idioms and Slang Expressions
Human language is rife with idioms and slang expressions that don’t follow literal interpretations. LLMs are often trained on vast datasets that encompass these linguistic nuances. However, if an LLM fails to grasp the underlying meaning of these expressions, it may stumble into the realm of hallucination when it encounters them in user prompts.
4. Lack of Context
Context is king when it comes to generating coherent and accurate responses. Imagine asking an LLM to write a story about a dog, but it has no knowledge of what a dog is. In such a scenario, the LLM might conjure a narrative that is factually incorrect or downright nonsensical. The absence of context can thus be a fertile breeding ground for AI hallucination.
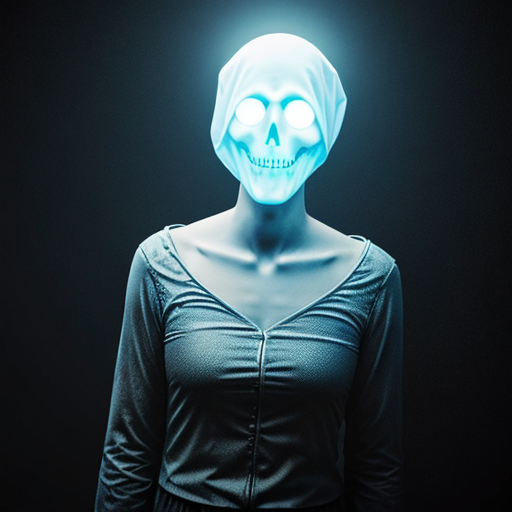
Preventing the Specter of AI Hallucination
Addressing the issue of AI hallucination requires a multi-pronged approach to ensure the reliability and trustworthiness of AI-generated content. Here are some strategies to consider:
1. Utilize a Large and Diverse Training Dataset
The cornerstone of preventing AI hallucination is the quality and quantity of the data used for training. Employing a large and diverse training dataset that mirrors the complexities of the real world can significantly reduce the likelihood of hallucinatory outputs. By exposing the AI model to a broad spectrum of information, you equip it to produce more accurate and context-aware responses.
2. Apply Regularization Techniques
Regularization techniques play a crucial role in preventing overfitting, which is a major contributor to AI hallucination. By applying these techniques during the training process, AI developers can help the model generalize better to new data and minimize the risk of generating false information. Regularization serves as the steadying hand that guides the AI’s creativity and ensures it stays grounded in reality.
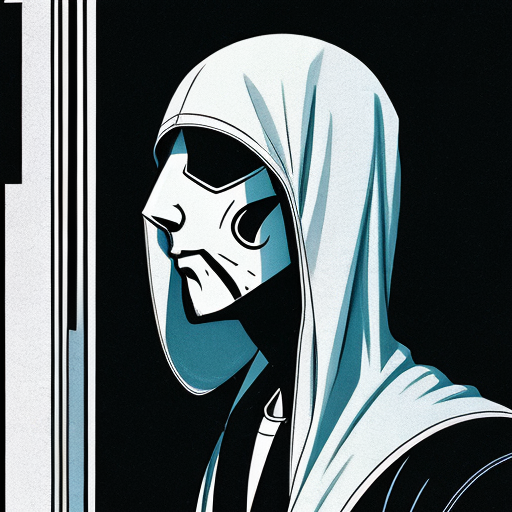
3. Train LLMs on Multiple Datasets
Diversification of training data is key to enhancing the robustness of LLMs. By training these models on multiple datasets from various sources and domains, we can reduce their vulnerability to hallucination. This approach ensures that LLMs are not overly influenced by any one dataset, thus promoting a more balanced and reliable generation of content.
4. Provide Adequate Context
To prevent AI from veering into the territory of hallucination, it’s imperative to provide it with sufficient context. When formulating prompts or requests for LLMs, developers should ensure that the AI has access to the necessary background information. A well-informed AI is less likely to wander into the realm of falsehoods and fabrications.
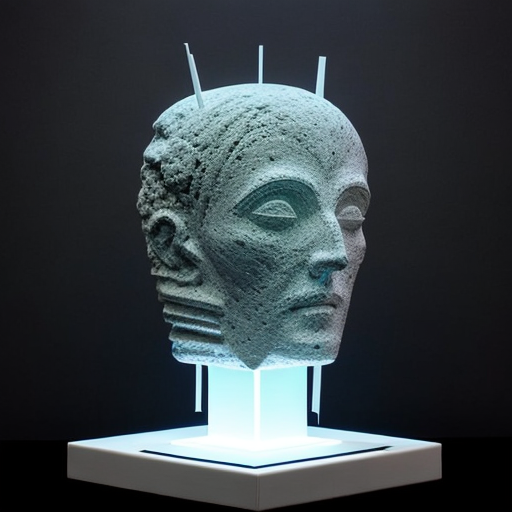
The Broader Implications of AI Hallucination
AI hallucination is not merely an esoteric concern for AI researchers; it carries profound implications for society at large. Here are some of the far-reaching consequences:
1. Misinformation and Disinformation
One of the most immediate and tangible risks posed by AI hallucination is the spread of misinformation and disinformation. In a world where AI-generated content is increasingly prevalent, the inadvertent or deliberate dissemination of false information could have severe consequences. This could impact everything from news reporting to academic research and public discourse.
2. Deceptive Media
AI hallucination has the potential to fuel the creation of deceptive media, such as deepfakes. These sophisticated manipulations of audio and video can convincingly depict individuals saying or doing things they never did. This could be exploited for various malicious purposes, including character assassination, political manipulation, and fraud.
3. Erosion of Trust
As AI-driven content becomes more integrated into our lives, the erosion of trust in information sources becomes a pressing concern. If users cannot rely on AI-generated responses to be accurate and reliable, they may become increasingly skeptical of AI-driven technologies and the information they provide.
4. Ethical and Legal Dilemmas
AI hallucination raises complex ethical and legal questions. Who bears responsibility when AI generates false information or deceptive content? How can we ensure accountability in a world where AI is responsible for generating vast amounts of content? These dilemmas require careful consideration and the development of regulatory frameworks.
Conclusion
AI hallucination is a double-edged sword in the realm of artificial intelligence. While LLMs have shown remarkable capabilities in generating human-like text and responses, the risk of generating false or hallucinatory information looms large. To harness the potential of AI without succumbing to its pitfalls, it is imperative to address the root causes of hallucination and implement robust prevention strategies.
As society navigates the evolving landscape of AI, the consequences of hallucination, from misinformation and deceptive media to the erosion of trust and ethical quandaries, must be reckoned with. It is only through a concerted effort, combining technical innovation, responsible AI development, and a commitment to ethical AI usage, that we can hope to mitigate the risks of AI hallucination and unlock the full potential of artificial intelligence for the betterment of humanity.